A team led by scientsts in the Baker lab has combined recent advances in evolutionary analysis and deep learning to build three-dimensional models of how most proteins in eukaryotes interact. This breakthrough has significant implications for understanding the biochemical processes that are common to all animals, plants, and fungi. This open-access work appears in Science.
“To really understand the cellular conditions that give rise to health and disease, it’s essential to know how different proteins in a cell work together. In this paper, we provide detailed information on protein interactions for nearly every core process in eukaryotic cells. This includes over a hundred interactions that have never been seen before.”
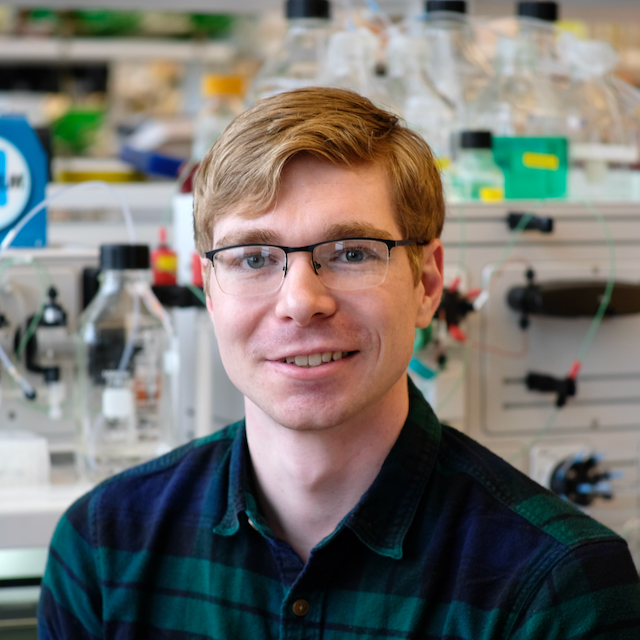
Proteins are the workhorses of all cells, but they rarely act alone. Different proteins often must fit together to form precise “complexes” that carry out specific tasks, including reading genes, digesting nutrients, and responding to signals from neighboring cells and the outside world. When protein complexes malfunction, disease can result.
“This work shows that deep learning can now generate real insights into decades-old questions in biology —not just what a particular protein looks like, but also which proteins come together to interact,” said senior author Qian Cong, an assistant professor in the department of biophysics at the University of Texas Southwestern Medical Center.
To exhaustively map the interactions that give rise to protein complexes, a team of structural biologists from UW Medicine, University of Texas Southwestern Medical Center, Harvard University, and other several institutions examined all known gene sequences in yeast. Using advanced statistical analyses, they identified pairs of genes that naturally acquire mutations in a linked fashion. They reasoned that such shared mutations are a sign that the proteins encoded by the genes must physically interact.
The researchers also used new deep-learning software to model the three-dimensional shapes of these interacting proteins. RoseTTAFold, invented at UW Medicine, and AlphaFold, invented by the Alphabet subsidiary DeepMind, were both used to generate hundreds of detailed pictures of protein complexes.
“As computer methods become more powerful, it is easier than ever to generate large amounts of scientific data, but to make sense of it still requires scientific experts,” said senior author David Baker, director of the Institute for Protein Design. “So we recruited a village of expert biologists to interpret our 3D protein models. This is community science at its best.”
The hundreds of newly identified protein complexes provide rich insights into how cells function. For example, one complex contains the protein RAD51, which is known to play a key role in DNA repair and cancer progression in humans. Another includes the poorly understood enzyme glycosylphosphatidylinositol transamidase, which has been implicated in neurodevelopmental disorders and cancer in humans. Understanding how these and other proteins interact may open the door to the development of new medications for a wide range of health disorders.
The protein structures generated in this work are available to download from the ModelArchive. The authors thank and remember John Westbrook at the Protein Data Bank for his support in establishing formats and software code to allow efficient deposition of the models into the archive; John sadly passed during the preparation of this manuscript.
The project was led by Ian Humphreys, Aditya Krishnakumar, and Minkyung Baek at UW Medicine as well as Jimin Pei at the University of Texas Southwestern Medical Center. Collaborating institutions include UW Medicine, UT Southwestern, Harvard University, Wayne State University, Cornell University, MRC Laboratory of Molecular Biology, Memorial Sloan Kettering Cancer Center, Gerstner Sloan Kettering Graduate School of Biomedical Sciences, Fred Hutchinson Cancer Research Center, Columbia University, University of Würzburg, St Jude Children’s Research Hospital, FIRC Institute of Molecular Oncology, and Istituto di Genetica Molecolare, Consiglio Nazionale delle Ricerche.
This work was supported by Microsoft, Amgen, Southwestern Medical Foundation, The Washington Research Foundation, Howard Hughes Medical Institute, National Science Foundation (DBI 1937533), National Institutes of Health (R35GM118026, R01CA221858, R35GM136258, R21AI156595), UK Medical Research Council (MRC_UP_1201/10), HHMI Gilliam Fellowship, the Deutsche Forschungsgemeinschaft (KI-562/11-1, KI-562/7-1), AIRC investigator and the European Research Council Consolidator (IG23710 and 682190), Defense Threat Reduction Agency (HDTRA1-21-1-0007), Cancer Prevention and Research Institute of Texas (RP210041), and National Energy Research Scientific Computing Center.