This advance could allow scientists to create cheaper alternatives to antibodies for disease detection and treatment.
This week we report in Nature an AI-enabled advance in biotechnology with implications for drug development, disease detection, and environmental monitoring. Using a combination of traditional and deep learning based molecular design approaches, we’ve created proteins that bind with exceptionally high affinity and specificity to a variety of challenging biomarkers, including human hormones. Notably, we achieved what we believe to be the highest binding affinity ever reported between a computer-generated biomolecule and its target.
AI-enabled protein design software in action. Beginning with a desired binding target (pink) and a cloud of disconnected amino acids, RFdiffusion iteratively sculpts a new protein structure that cradles the target peptide. At the end, ProteinMPNN assigns amino acid side chains to the new protein structure, yielding a complete protein molecule. Laboratory tests reveal this protein binds its target with the highest affinity ever reported to date for a computer-generated protein without any experimental optimization.
This project was led by Baker Lab members Susana Vazquez-Torres, Preetham Venkatesh, and Phil Leung, PhD. It included collaborators from UW Medicine and the University of Copenhagen, as well as from our Core R&D Labs.
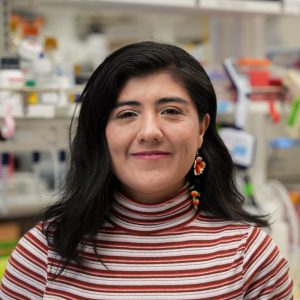
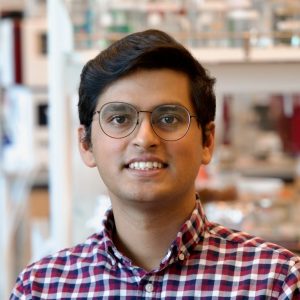
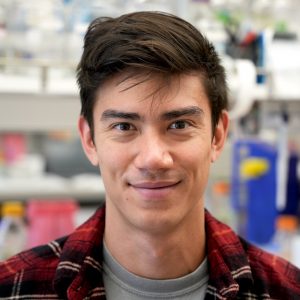
Targeting helical peptides
The team set out to create proteins that could bind to glucagon, neuropeptide Y, parathyroid hormone, and other helical peptide targets. Such molecules, crucial in biological systems, are especially challenging for drugs and diagnostic tools to recognize as they often lack stable molecular structures. Antibodies can be used to detect some of these targets but are often costly to produce and have limited shelf lives.
“There are many diseases that are difficult to treat today simply because it is so challenging to detect certain molecules in the body. As tools for diagnosis, designed proteins may offer a more cost-effective alternative to antibodies,” explains Venkatesh.
The role of generative AI
The study introduces a novel way of using RFdiffusion, a generative model for creating new protein shapes, in conjunction with the sequence-design tool ProteinMPNN. Developed in the Baker Lab, these programs allow scientists to create functional proteins more efficiently than ever before. By combining these tools in new ways, the team was able to create binding proteins by using limited target information, such as a peptide’s amino acid sequence alone.
“We’re witnessing an exciting era in protein design, where advanced artificial intelligence tools, like the ones featured in our study, are accelerating the improvement of protein activity. This breakthrough is set to redefine the landscape of biotechnology,” notes Vazquez-Torres.
Measuring binding in the lab
In collaboration with the Joseph Rogers Lab at the University of Copenhagen and Andrew Hoofnagle Lab at UW Medicine, we conducted laboratory tests to validate the new biodesign methods.
Mass spectrometry was used to detect designed proteins that bind to low-concentration peptides in human serum, thereby demonstrating the potential for sensitive and accurate disease diagnostics. Additionally, the proteins were found to retain their target binding abilities despite harsh conditions including high heat, a crucial attribute for real-world application.
From binders to biosensors
With improved methods for creating binding proteins in place, the team turned to the challenge of designing new biosensors. To make sensors that could detect parathyroid hormone (PTH), we grafted a high-affinity PTH binder into our previously reported lucCage biosensor system. The best-performing biosensor lit up when mixed with PTH, displaying a 21-fold increase in bioluminescence.
“The ability to design proteins with such high affinity and specificity opens up a world of possibilities, from new disease treatments to advanced diagnostics,” concludes senior author and Institute director David Baker.
Funding
This work was supported by the National Institutes of Health (T1D U01 DK121289, U19 AG065156, K99EB031913, P30 GM124165), National Science Foundation (EF-2021552), Department of Energy (BER-ERCAP0022018; DE-AC02-06CH11357), European Molecular Biology Organization (ALTF 292-2022), Washington State General Operating Fund, Amgen, Audacious Project, AWS, Bill and Melinda Gates Foundation (INV-010680), Donald and Jo Anne Petersen, Howard Hughes Medical Institute, Microsoft, Novo Nordisk Foundation (NNF19OC0054441), Open Philanthropy Project, and Partnership for Clean Competition.